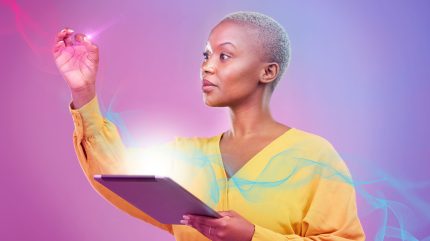
Artificial Intelligence (AI) has become a cornerstone of modern technology, influencing decisions in critical areas such as law enforcement, healthcare, hiring, and more.
However, as AI systems become more prevalent, a disturbing trend has emerged: AI often exhibits racist behaviour.
This raises the question: Why is AI racist? This article delves into the root causes of racial bias in AI, its consequences, and what can be done to mitigate this problem.
Understanding AI and its origins
The role of data in AI
At its core, AI is powered by data. Machine learning algorithms, a subset of AI, are trained on large datasets to recognise patterns, make decisions, and predict outcomes.
These datasets are a reflection of the real world, encompassing information from various sources—images, text, audio, and more.
The AI’s ability to perform its tasks is directly proportional to the quality and diversity of the data it is trained on.
Historical and societal biases in data
The problem begins with the data itself. Society has a long history of racial discrimination, and this is reflected in the data collected over time.
Historical biases, stereotypes, and systemic racism are all embedded in the datasets used to train AI models.
For example, law enforcement data that disproportionately targets minority communities can lead to predictive policing algorithms that continue to unfairly target these groups.
Similarly, hiring data that favors certain racial groups can perpetuate discrimination in AI-driven recruitment tools.
The manifestation of racial bias in AI
Facial recognition systems
One of the most visible examples of racial bias in AI is found in facial recognition systems.
Numerous studies have shown that these systems are far less accurate in identifying people of colour, particularly Black and Asian individuals.
This is largely due to the underrepresentation of these groups in the training datasets, leading to higher error rates and, in some cases, wrongful arrests or misidentifications.
Predictive policing
AI systems used in law enforcement, such as predictive policing algorithms, are designed to predict where crimes are likely to occur or who is likely to commit them.
However, if the underlying data reflects biased policing practices—such as over-policing in minority neighbourhoods—the AI will learn and reinforce these biases.
This creates a feedback loop where certain communities are continuously targeted, perpetuating racial injustice.
Bias in healthcare
AI in healthcare holds the promise of personalised medicine and improved diagnostics, but racial bias can undermine these benefits.
For example, algorithms used to predict patient outcomes may not perform as well for minority groups if they are underrepresented in the data.
This can lead to misdiagnoses or inadequate treatment for these populations, exacerbating existing health disparities.
Why Is AI racist?
Inadequate representation
One of the primary reasons AI exhibits racial bias is the inadequate representation of minority groups in the training data.
When AI systems are trained predominantly on data from one racial group, they struggle to generalise to others.
This lack of diversity in data leads to models that are inherently biased and less accurate for underrepresented groups.
Implicit human bias
AI systems are often trained using data labelled by humans, and human biases inevitably seep into this process.
If the individuals labelling the data hold subconscious or conscious biases, these biases will be reflected in the AI’s output.
For instance, if a dataset is labelled with biased assumptions about race and criminality, the AI will learn to associate certain racial groups with criminal behaviour, even if there is no factual basis for such an association.
The complexity of bias in AI
Addressing racial bias in AI is not simply a matter of removing biased data.
Bias in AI is multifaceted and can be influenced by a range of factors, including the algorithms used, the data collection methods, and the way the AI is deployed.
Moreover, attempts to correct one type of bias can inadvertently introduce new forms of bias, making the problem even more complex.
The consequences of racially biased AI
Reinforcing systemic racism
When AI systems perpetuate racial biases, they reinforce existing systemic racism.
For example, biased AI in the criminal justice system can lead to disproportionate targeting and incarceration of minority communities, exacerbating the cycle of poverty and disenfranchisement.
Erosion of trust
The prevalence of racial bias in AI also erodes public trust in these technologies.
Communities that are disproportionately affected by biased AI may become distrustful of AI-driven decisions, whether in policing, healthcare, or employment.
This lack of trust can hinder the adoption of AI and limit its potential benefits for society.
Legal and ethical implications
There are significant legal and ethical implications associated with racially biased AI.
Discrimination based on race is illegal in many jurisdictions, and AI systems that perpetuate such discrimination could face legal challenges.
Additionally, there are ethical concerns about the fairness and justice of AI systems that disadvantage certain racial groups.
Addressing racial bias in AI
Improving data diversity
To reduce racial bias in AI, it is crucial to improve the diversity of the training data.
This means actively seeking out and including data from underrepresented groups, ensuring that AI systems are trained on a broad and representative dataset.
This can help create models that are fairer and more accurate across different racial groups.
Implementing bias mitigation techniques
Developers can also implement bias mitigation techniques during the AI development process.
These techniques include reweighting training data to account for underrepresented groups, applying fairness constraints to the algorithms, and using adversarial debiasing methods to reduce bias in the model’s output.
Promoting transparency and accountability
Transparency and accountability are key to addressing racial bias in AI.
AI developers and companies need to be open about how their systems work, what data they use, and the potential biases that may exist.
Regular audits and the involvement of independent oversight bodies can help ensure that AI systems are fair and do not perpetuate racial discrimination.
Ethical AI development
Finally, promoting ethical AI development practices is essential.
This involves considering the societal impacts of AI systems, prioritising fairness and equity, and ensuring that AI benefits all members of society, regardless of race.
Exploring potential solutions to AI racism
1. Inclusive data collection practices
A significant step toward mitigating racial bias in AI is adopting inclusive data collection practices.
This involves not just increasing the quantity of data from underrepresented groups but ensuring that this data captures the diversity of experiences within these groups.
For example, in healthcare, this could mean collecting data that accounts for different ways diseases present in various racial groups.
Moreover, data collection should be conducted with sensitivity to cultural contexts, avoiding the perpetuation of stereotypes.
2. Algorithmic fairness and testing
AI developers need to prioritise fairness in their algorithms from the outset.
This can be achieved by designing algorithms that are explicitly tested for bias across different demographic groups.
Fairness-aware machine learning techniques, such as ensuring equal error rates across groups, can help create more equitable outcomes.
Regular testing and validation of algorithms against diverse datasets are also essential to ensure ongoing fairness as models are updated and retrained.
3. Human-in-the-loop approaches
Incorporating human oversight in AI decision-making processes can help mitigate the impact of biased AI outputs.
Human-in-the-loop approaches allow for the review and correction of AI decisions, particularly in high-stakes areas such as law enforcement and healthcare.
This approach can provide a critical check on AI systems, ensuring that biased outputs are identified and addressed before they cause harm.
4. Regulation and policy development
Governments and regulatory bodies play a crucial role in addressing racial bias in AI. There is a growing need for clear regulations that mandate fairness and accountability in AI systems.
This includes requirements for transparency in how AI systems are trained, tested, and deployed, as well as the establishment of standards for evaluating AI fairness.
Policies that encourage or require the use of diverse data and the regular auditing of AI systems can help ensure that these technologies serve all communities equitably.
5. Ethical AI frameworks and standards
Developing and adopting ethical AI frameworks is another essential solution.
These frameworks should outline the principles and standards for responsible AI development, including fairness, accountability, transparency, and inclusivity.
By adhering to these standards, AI developers can ensure that their systems are designed and deployed in ways that minimise racial bias and promote justice.
6. Education and awareness
Raising awareness about the potential for racial bias in AI is crucial for both developers and the general public.
Educating AI professionals about the ethical implications of their work can lead to more thoughtful and responsible AI development.
Public awareness campaigns can also help users of AI systems understand the limitations and potential biases of these technologies, empowering them to demand fairer AI.
7. Collaboration across sectors
Finally, addressing racial bias in AI requires collaboration across various sectors, including technology, academia, government, and civil society.
Multidisciplinary teams that bring together experts in AI, ethics, law, and social sciences can work together to develop solutions that are both technically robust and socially responsible.
By fostering collaboration, stakeholders can share best practices, create innovative solutions, and build AI systems that are fair and equitable.
The takeaway
AI has the potential to transform society for the better, but only if it is developed and deployed in a way that is fair and equitable. The racial bias present in many AI systems today is a reflection of broader societal issues and historical injustices.
By understanding the root causes of this bias and taking proactive steps to address it, we can create AI systems that are not only powerful but also just and inclusive.
Only then can AI truly serve the needs of all people, regardless of their race or ethnicity.
The solutions outlined above—ranging from improving data diversity and implementing fairness testing, to fostering collaboration and developing ethical frameworks—are crucial steps toward ensuring that AI systems do not perpetuate racial discrimination.
As AI continues to shape the future, it is imperative that we work collectively to ensure that it advances equity and justice for all.